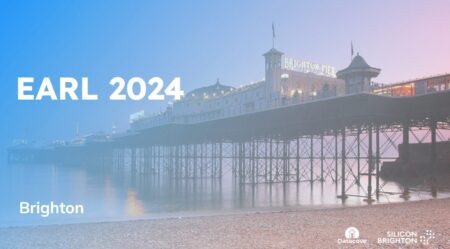
See the full agenda and book your tickets today for EARL 2024 and key in RBTBZO at the checkout for a limited (while stocks last) 10% saving on your tickets as a special for R bloggers readers.
The Enterprise Applications of the R Language (EARL) Conference is a cross-sector tech conference focusing on the commercial use of the R programming language. Join us on 3rd – 5th September 2024 in Brighton for its 10th year as we hear from some of the world’s leading practitioners, consultants and industry experts in R, Python and data science.
Yes, you read that correctly! EARL has long been a cornerstone for professionals across industries who leverage R for real-world data challenges, but this year, we’re expanding our horizons by integrating Python. This convergence promises a more comprehensive view of modern data science, bridging statistical expertise with advanced computational capabilities.
New city, new languages – and new hosts too, with Brighton based data and analytics consultancy Datacove and Brighton’s tech sector hosts, Silicon Brighton taking over from the wonderful team at Ascent who have made EARL the event it is today.
As if that wasn’t enough to get you excited, our line-up of speakers includes the one and only Hadley Wickham! Joining him are Christel Swift from the BBC, Steph Locke from Microsoft, and renowned statistician and author Andy Field from the University of Sussex, each offering their unique insights on the industry today – and where we are headed tomorrow!
The conference agenda spans three days of workshops, keynotes, and talks featuring 40+ speakers from a diverse range of diverse and markets. It’s an unparalleled opportunity to learn, network, and engage with leading minds in both R and Python.
EARL 2024 wouldn’t be possible without the generous support of sponsors Posit, Ascent, and the R Consortium – and maybe you too! If you would like to showcase your brand in front of more than 200 of the world’s leading tech professionals, there are still sponsorship and advertising opportunities available. More details can be found on the EARL website, below.
We are excited to bring EARL 2024 to Brighton, a vibrant hub of culture, technology and innovation, and can’t wait to welcome you to what promises to be the most dynamic conference yet. Join us at The Grand Hotel, an elegant and historic venue located on the city’s picturesque seafront, blending classic charm with modern comforts. You’ll be treated to a delicious buffet lunch on both days of the conference, prepared by some of the city’s finest chefs – with tea and coffee also available throughout. Then cross the road for a memorable evening event at the British Airways i360, with stunning panoramic views of the city and coastline from the world’s tallest moving observation tower.
See the full agenda and book your tickets today for EARL 2024 and key in RBTBZO at the checkout for a limited (while stocks last) 10% saving on your tickets as a special for R bloggers readers.
See you in Brighton!