The Herfindahl-Hirschman Index (HHI) is a widely used measure of concentration in a variety of fields including, business, economics, political science, finance, and many others. Though simple to calculate (summed squared market shares of firms/actors in a single market/space), calculation of the HHI can get onerous, especially as the number of firms/actors increases and the time period grows. Thus, I decided to write a package aimed at streamlining and simplifying calculation of HHI scores. The package, hhi, calculates the concentration of a market/space based on a supplied vector of values corresponding with shares of all individual firms/actors acting in that space. The package is available on CRAN.
The purpose of this blog post is to provide a quick overview of the package’s two key functions: hhi (calculation) and plot_hhi (visualization).
Calculating HHI Scores
As the package is intended for simple, intuitive usage, the function requires only the name of the data frame and then the name of the variable corresponding with the market shares in quotation marks. With these placed directly in the command, calling the function hhi will generate the HHI score based on the values supplied, following the basic form,
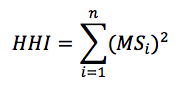
Consider a simple application calculating the HHI for the men’s footwear market in the United States in 2017 (see and download the data file, “footwear.txt”, from my GitHub repo). Using market share data for every men’s footwear company operating in the U.S. in 2017 from Euromonitor Passport, we can calculate this market’s HHI with the following code:
# First, install the "hhi" package, then load the library install.packages("hhi") library(hhi) # Next, read in data: US Men's Footwear Company Market Shares, 2012-2017 footwear = read.table(".../footwear.txt") # Now, call the "hhi" command to calculate HHI for 2017 hhi(footwear, "ms.2017") # first the df, then the shares variable in quotes
Calling the function hhi gives us an HHI index value for men’s footwear in the U.S. in 2017 of 2009.25. You can corroborate this output manually by squaring each market share corresponding with each company in the data file in the year 2017, and then summing over each firm’s squared market share.Often, the HHI is used as a measure of competition, with 10,000 equaling perfect monopoly (100^2) and 0.0 equaling perfect competition. As such, we can see that the U.S. men’s footwear industry in 2017 seems relatively competitive. Yet, to say anything substantive about the men’s U.S. footwear market, we really need a comparison of HHI scores for this market over time. This is where the second command comes in.
Visualizing HHI Time Series
The second key function in the package, plot_hhi, is a plotting feature allowing for quick and simple visualization of a time series of HHI scores. Usage is similarly straightforward, requiring only the name of the data frame, the name of the variable corresponding with the time indicator in quotation marks, and then the name of the variable corresponding with the market shares also in quotation marks. The package leverages ggplot2 to provide a visual rendering of the supplied vector of HHI values over the specified range of time. The function supports any measure of time, such as, years, quarters, months, etc. Note that plot_hhi is a relatively inflexible function meant for quick visual rendering of a vector of HHI scores over a period of time. For bigger and formal projects, users are advised to generate original plots with other plotting functions and packages beyond hhi to allow for greater flexibility in customizing visual output according to specific needs.Let’s return to our men’s U.S. footwear example to see how the function works in practice. First, we need to calculate the HHI scores for each year in the data file (2012-2017), and store those as objects to make a data frame of HHI scores corresponding to individual years. Then, we simply call the plot_hhi command and generate a simple, pleasing plot of HHI scores over time. This will give us a much better sense of how our 2017 HHI score above compares with other years in this market. See the code below, followed by the output.
# First, calculate and store HHI for each year in the data file (2012-2017) hhi.12 = hhi(footwear, "ms.2012") hhi.13 = hhi(footwear, "ms.2013") hhi.14 = hhi(footwear, "ms.2014") hhi.15 = hhi(footwear, "ms.2015") hhi.16 = hhi(footwear, "ms.2016") hhi.17 = hhi(footwear, "ms.2017") # Combine and create df for plotting hhi = rbind(hhi.12, hhi.13, hhi.14, hhi.15, hhi.16, hhi.17) year = c(2012, 2013, 2014, 2015, 2016, 2017) hhi.data = data.frame(year, hhi) # Finally, generate HHI time series plot using the "plot_hhi" command plot_hhi(hhi.data, "year", "hhi")
These lines of code will give us the following plot of HHI scores for each year in the data set.
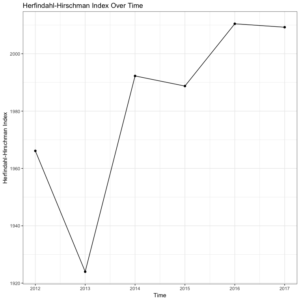
Interestingly, the men’s U.S. footwear industry seems to be getting slightly less competitive (higher HHI scores) from 2012 to 2017, on average. To say anything substantive about this trend, though, would obviously require more sophisticated methods as well as a longer time series. Yet, the value of the hhi package is allowing for quick calculation and visualization of HHI scores over time. You can download the package from CRAN or directly from the package installation context in RStudio. And as always, if you have any questions or find any bugs requiring fixing, please feel free to contact me.
As a final note, here are a few references for further reading on the HHI and its original calculation and intuition:Herfindahl, Orris C. 1950. “Concentration in the steel industry.” Ph.D. dissertation, Columbia University.
Hirschman, Albert O. 1945. “National power and structure of foreign trade.” Berkeley, CA: University of California Press.
Rhoades, Stephen A. 1993. “The herfindahl-hirschman index.” Federal Reserve Bulletin 79: 188.
Thanks and enjoy!
Hi there, this is really useful for me — thanks. I’m an R newb so hoping that this question makes sense!
Is there any way that you can add several vectors into the argument? How I’m reading it now is that R takes the vector and applies the HHI formula to each element within it, to create the score. My data are structured in a different way, as it is based on locality. So I have 600 areas (each one is a case in a column) then 11 companies (each one is a vector/row), meaning the elements are populated with the market share for that company in that area to make a dataframe. I hope I’ve explained that okay. I want to create a new vector that holds the HHI score for each area. Is that possible with this package?
Hi Hannah – so glad the package is useful for you! I think your question makes sense, but I have some sample code I would like to share to hopefully help out a bit more. Would you mind sending me this question via email so we can have a more extended discussion offline to avoid many back and forth comments on this post? You can find my email at my website: http://www.philipdwaggoner.com. Thanks!