I stumbled upon the Emotion API when I read a post by Kan Nishida from exploritory.io, which analyzed the facial expressions of Trump and Clinton during a presedential debate last year. However, that tutorial (like many others) no longer works, since it used the old Emotion API.
Lately I needed a tool for an analysis I did at work on the facial expressions in TV commercials. I had a list of videos showing faces, and I needed to code these faces into emotions.
I noticed that Microsft still offers a simpler “face API”. This API doesn’t work with videos, it only runs on still images (e.g. jpegs). I decided to use it and here are the results (bottom line – you can use it for videos after some prep work).
By the way, AWS and google have similar APIs (for images) called: Amazon Rekognition (not a typo) and Vision API respectively.
Here is guide on how to do a batch analysis of videos and turn them into a single data frame (or tibble) of emotions which are displayed in the videos per frame.
To use the API you need a key – if you don’t already have it, register to Microsoft’s Azure API and register a new service of type Face API. You get an initial “gratis” credit of about $200 (1,000 images cost $1.5 so $200 is more than enough).
Preparations
First, we’ll load the packages we’re going to usehttr
to send our requests to the server, and tidyverse
(mostly for ggplot
, dplyr
, tidyr
, tibble
). Also, lets define the API access point we will use, and the appropriate key. My face API service was hosted on west Europe (hence the URL starts with westeurope.api.
# ==== Load required libraries ====
library(tidyverse)
library(httr)
# ==== Microsoft's Azure Face API ====
end.point <- "https://westeurope.api.cognitive.microsoft.com/face/v1.0/detect"
key1 <- "PUT YOUR SECRET KEY HERE"
To get things going, lets check that the API and key works. We’ll send a simple image (of me) to the API and see what comes out.
sample.img.simple <- POST(url = end.point,
add_headers(.headers = c("Ocp-Apim-Subscription-Key" = key1)),
body = '{"url":"http://www.sarid-ins.co.il/files/TheTeam/Adi_Sarid.jpg"}',
query = list(returnFaceAttributes = "emotion"),
accept_json())
This is the simplest form of the API, which returns only emotions of the faces depicted in the image. You can ask for a lot of other features by setting them in the query parameter. For example, to get the emotions, age, gender, hair, makeup and accessories use returnFaceAttributes = "emotion,age,gender,hair,makeup,accessories"
. Here’s a full documentation of what you can get.
Later on we’ll change the body parameter at the
POST
from a json which contains a URL to a local image file which will be uploaded to Microsoft’s servers.For now, lets look at the response of this query (notice the reference is for the first identified face
[[1]]
, for an image with more faces, face i
will appear in location [[i]]
).
as_tibble(content(sample.img.simple)[[1]]$faceAttributes$emotion) %>% t()
## [,1] ## anger 0.001 ## contempt 0.062 ## disgust 0.002 ## fear 0.000 ## happiness 0.160 ## neutral 0.774 ## sadness 0.001 ## surprise 0.001You can see that the API is pretty sure I’m showing a neutral face (0.774), but I might also be showing a little bit of happiness (0.160). Anyway these are weights (probabilities to be exact), they will always sum up to 1. If you want a single classification you should probably choose the highest weight as the classified emotion. Other results such as gender, hair color, work similarly.
Now we’re ready to start working with videos. We’ll be building a number of functions to automate the process.
Splitting a video to individual frames
To split the video file into individual frames (images which we can send to the API), I’m going to (locally) use ffmpeg by calling it from R (it is run externally by the system – I’m using windows for this). Assume thatfile.url
contains the location of the video (can be online or local), and that id.number
is a unique string identifier of the video.
movie2frames <- function(file.url, id.number){
base.dir <- "d:/temp/facial_coding/"
dir.create(paste0(base.dir, id.number))
system(
paste0(
"ffmpeg -i ", file.url,
" -vf fps=2 ", base.dir,
id.number, "/image%07d.jpg")
)
}
The parameter fps=2
in the command means that we are extracting two frames per second (for my needs that was a reasonable fps res, assuming that emotions don’t change that much during 1/2 sec).Be sure to change the directory location (
base.dir
from d:/temp/facial_coding/
) to whatever you need. This function will create a subdirectory within the base.dir
, with all the frames extracted by ffmpeg. Now were ready to send these frames to the API.
A function for sending a (single) image and reading back emotions
Now, I defined a function for sending an image to the API and getting back the results. You’ll notice that I’m only using a very small portion of what the API has to offer (only the faces). For the simplicity of the example, I’m reading only the first face (there might be more than one on a single image).send.face <- function(filename) { face_res <- POST(url = end.point, add_headers(.headers = c("Ocp-Apim-Subscription-Key" = key1)), body = upload_file(filename, "application/octet-stream"), query = list(returnFaceAttributes = "emotion"), accept_json()) if(length(content(face_res)) > 0){ ret.expr <- as_tibble(content(face_res)[[1]]$faceAttributes$emotion) } else { ret.expr <- tibble(contempt = NA, disgust = NA, fear = NA, happiness = NA, neutral = NA, sadness = NA, surprise = NA) } return(ret.expr) }
A function to process a batch of images
As I mentioned, in my case I had videos, so I had to work with a batch of images (each image representing a frame in the original video). After splitting the video, we now have a directory full of jpgs and we want to send them all to analysis. Thus, another function is required to automate the use ofsend.face()
(the function we had just defined).
extract.from.frames <- function(directory.location){ base.dir <- "d:/temp/facial_coding/" # enter directory location without ending "/" face.analysis <- dir(directory.location) %>% as_tibble() %>% mutate(filename = paste0(directory.location,"/", value)) %>% group_by(filename) %>% do(send.face(.$filename)) %>% ungroup() %>% mutate(frame.num = 1:NROW(filename)) %>% mutate(origin = directory.location) # Save temporary data frame for later use (so not to loose data if do() stops/fails) temp.filename <- tail(stringr::str_split(directory.location, stringr::fixed("/"))[[1]],1) write_excel_csv(x = face.analysis, path = paste0(base.dir, temp.filename, ".csv")) return(face.analysis) }The second part of the function (starting from “# Save temporary data frame for later use…”) is not mandatory. I wanted the results saved per frame batch into a file, since I used this function for a lot of movies (and you don’t want to loose everything if something temporarily doesn’t work). Again, if you do want the function to save its results to a file, be sure to change
base.dir
in extract.from.frames
as well – to suit your own location.By the way, note the use of
do()
. I could also probably use walk()
, but do()
has the benefit of showing you a nice progress bar while it processes the data. Once you call this
results.many.frames <- extract.from.frames("c:/some/directory")
, you will receive a nice tibble that looks like this one:
## Observations: 796 ## Variables: 11 ## $ ..filename d:/temp/facial_coding/119/image00001.jpg, d:/temp... ## $ anger 0.000, 0.000, 0.000, 0.000, 0.000, 0.000, 0.000, 0... ## $ contempt 0.001, 0.001, 0.000, 0.001, 0.002, 0.001, 0.001, 0... ## $ disgust 0, 0, 0, 0, 0, 0, 0, 0, 0, 0, 0, 0, 0, 0, 0, 0, 0,... ## $ fear 0, 0, 0, 0, 0, 0, 0, 0, 0, 0, 0, 0, 0, 0, 0, 0, 0,... ## $ happiness 0.000, 0.000, 0.000, 0.000, 0.000, 0.000, 0.000, 0... ## $ neutral 0.998, 0.998, 0.999, 0.993, 0.996, 0.997, 0.997, 0... ## $ sadness 0.000, 0.000, 0.000, 0.001, 0.001, 0.000, 0.000, 0... ## $ surprise 0.001, 0.001, 0.000, 0.005, 0.001, 0.002, 0.001, 0... ## $ frame.num 1, 2, 3, 4, 5, 6, 7, 8, 9, 10, 11, 12, 13, 14, 15,... ## $ origin d:/temp/facial_coding/119, d:/temp/facial_coding/...
Visualization
Here is the visualization of the emotion classification which were detected in this movie, as a function of frame number.res.for.gg <- results.many.frames %>% select(anger:frame.num) %>% gather(key = emotion, value = intensity, -frame.num) glimpse(res.for.gg)
## Observations: 6,368 ## Variables: 3 ## $ frame.num <int> 1, 2, 3, 4, 5, 6, 7, 8, 9, 10, 11, 12, 13, 14, 15, 1... ## $ emotion <chr> "anger", "anger", "anger", "anger", "anger", "anger"... ## $ intensity <dbl> 0.000, 0.000, 0.000, 0.000, 0.000, 0.000, 0.000, 0.0...
ggplot(res.for.gg, aes(x = frame.num, y = intensity, color = emotion)) + geom_point()
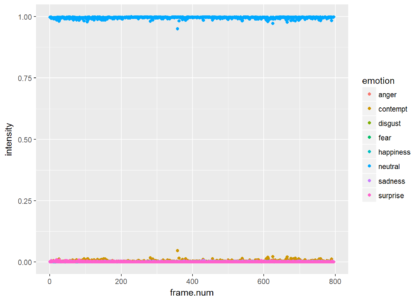
Since most of the frames show neutrality at a very high probability, the graph is not very informative. Just for the show, lets drop neutrality and focus on all other emotions. We can see that the is a small probability of some contempt during the video. The next plot shows only the points and the third plot shows only the smoothed version (without the points).
ggplot(res.for.gg %>% filter(emotion != "neutral"), aes(x = frame.num, y = intensity, color = emotion)) + geom_point()
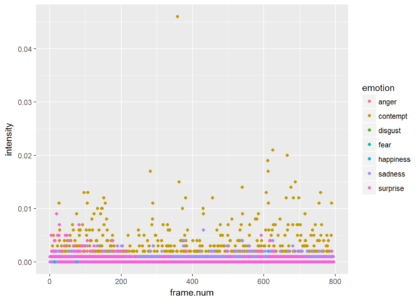
ggplot(res.for.gg %>% filter(emotion != "neutral"), aes(x = frame.num, y = intensity, color = emotion)) + stat_smooth()
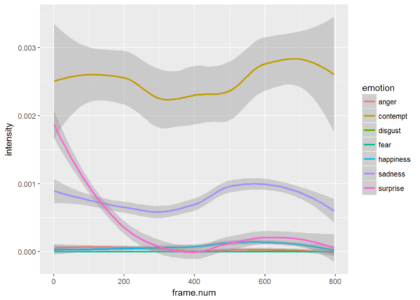
Conclusions
Though the Emotion API which used to analyze a complete video has been deprecated, the Face API can be used for this kind of video analysis, with the addition of splitting the video file to individual frames.The possibilities with the face API are endless and can fit a variety of needs. Have fun playing around with it, and let me know if you found this tutorial helpful, or if you did something interesting with the API.